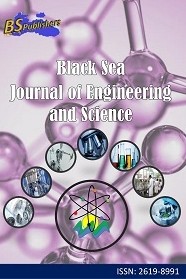
Black Sea Journal of Engineering and Science
Yazarlar: Müslüme MEMİŞ, Hasan ÖNDER
Konular:Mühendislik
Anahtar Kelimeler:Poisson regression,Generalized linear models,Maximum likelihood
Özet: The aim of many scientific studies is to explain relationships between response variable and explanatory variables with mathematical models and to acquire prudential predictions with these models. Poisson regression models are commonly usedfor analyzing the data based on counting processes. This study aimed to guide the researchers for determining appropriate Poisson regression estimation method (Poisson Maximum Likelihoodand Generalized Linear Model). In comparison of methods, artificial data were used with sample size of 100, 500 and 1000. It was concluded that there were no differences among parameter estimation methods in terms of goodness of fit. However, it was detected that generalized linear models method was more reliable than maximum likelihood method because maximum likelihood estimator produced high standard error for the parameters. In addition, generalized linear models were more reliable for small sample sizes because of estimated lover standard errors. As a result, it was suggested that generalized linear models should be used in Poisson regression analysis.