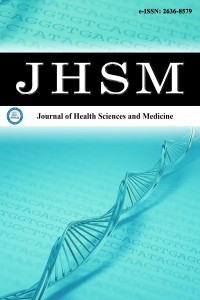
Journal of Health Sciences and Medicine
Yazarlar: ["Hakan YILDIRIM", "Ülkü ÇELİKER", "Sabiha GÜNGÖR KOBAT", "Sengul DOGAN", "Mehmet BAYĞIN", "Orhan YAMAN", "Türker TUNCER", "Murat ERDAĞ"]
Konular:-
DOI:10.32322/jhsm.1184981
Anahtar Kelimeler:Diabetic retinopathy,Fundus image processing,Biomedical image classification,Artificial intelligence
Özet: Aim: Fundus images are very important to diagnose some ophthalmologic disorders. Hence, fundus images have become a very important data source for machine-learning society. Our primary goal is to propose a new automated disorder classification model for diabetic retinopathy (DR) using the strength of deep learning. In this model, our proposed model suggests a treatment technique using fundus images. Material and Method: In this research, a new dataset was acquired and this dataset contains 1365 Fundus Fluorescein Angiography images with five classes. To detect these disorders automatically, we proposed a transfer learning-based feature engineering model. This feature engineering model uses pretrained MobileNetv2 and nested patch division to extract deep and exemplar features. The neighborhood component analysis (NCA) feature selection function has been applied to choose the top features. k nearest neighbors (kNN) classification function has been used to get results and we used 10-fold cross-validation (CV) to validate the results. Results: The proposed MobileNetv2 and nested patch-based image classification model attained 87.40% classification accuracy on the collected dataset. Conclusions: The calculated 87.40% classification accuracy for five classes has been demonstrated high classification accuracy of the proposed deep feature engineering model