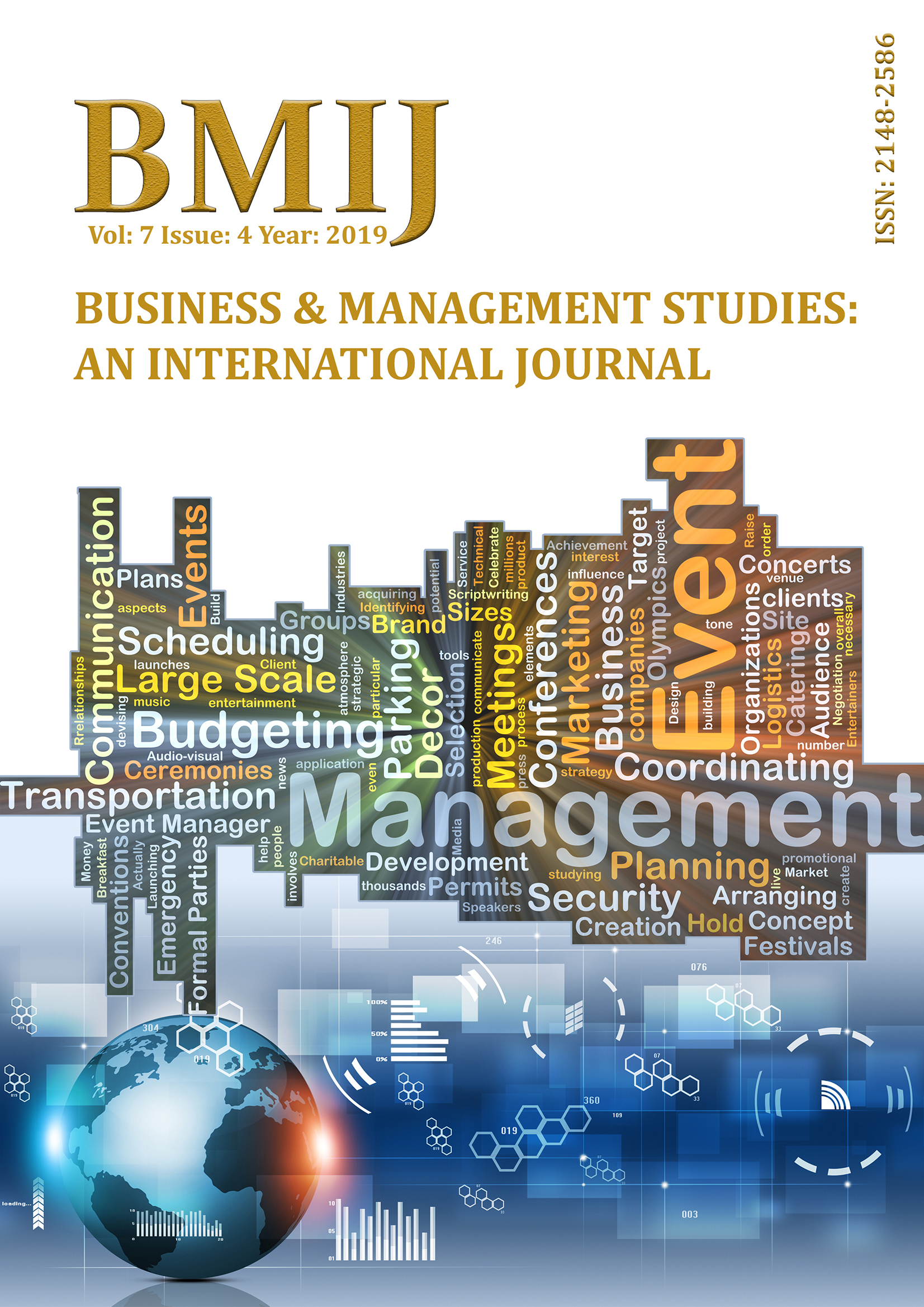
Business & Management Studies: An International Journal
Yazarlar: Melih BAŞKOL
Konular:-
Anahtar Kelimeler:Segmentasyon,Müşteri Veri Analizi,RFM,Uyum Analizi Segmentation Customer Data Analysis RFM Correspondence Analysis
Özet: INTRODUCTION Segmentation is a strategy that includes dividing a more extensive market into small groups of consumers with distinct needs, characteristics or behaviours that may require different marketing programs, according to these characteristics and behavioural similarities. Segmentation helps companies learn more about consumer preferences and needs, so companies can adapt different policies for targeted segments to increase consumer satisfaction and company revenues. The development of market segmentation theory has been linked to the accessibility of marketing data, improvements in analytical techniques, and segmentation method advancement (Liu, Liao, Huang & Liao, 2019). In this respect, customer segmentation is used as a marketing differentiation tool that enables companies to understand their customers and create different strategies (Silva, Varela, Borrero López & Rojas Millán, 2019). Target customer selection has been one of the most critical issues in customer-based marketing. Therefore, the key to finding profitable or potentially profitable customers is creating value via the customers’ help. Today, the ability to identify profitable, loyal and long-term customers is the most crucial success factor for customer-oriented organizations. To arrive at winning strategies, business owners have to look for an appropriate approach to identify potential customers and attract them as much as possible (Zalaghi and Abbasnejad Varzi, 2014). In this respect, the most effective and easiest model for identifying valuable consumers is the RFM model (Tsao, Raj & Yu, 2019). Several techniques are suggested in the literature for performing customer segmentation, among which clustering is the most commonly used method. However, the RFM model is effectively used to understand and analyze customer behaviour characteristics. Its simplicity and applicability are among the well-known and most widely used methods for customer analysis and segmentation (Peker, Kocyigit, & Eren, 2017). The primary purpose of this study is to reveal that customer segmentation can be done better with the help of RFM analysis and how these customer segments relate to the products they purchase. In this direction, first of all, the concepts of segmentation, RFM and correspondence analysis, which are critical competitive tools in the retail sector, have been tried to be explained based on the literature. The study aimed to divide customers into segments with RFM analysis based on customer records and name each customer segment. It is also among the study’s aims to show the retailers that they can examine the customer segments in more detail by visualizing the brand choices by determining which brands in a particular product group are closer to detailed customer segments. RESEARCH METHODOLOGY In the first part of the research, the store customers’ loyalty dimensions were determined by RFM analysis. Past purchases of customers are highly effective in predicting future purchasing behaviour. Thus, companies can quickly determine with which customers they are worth contacting in the future through RFM (Mohammadian & Makhani, 2019). The data have been cleared in order to make the necessary arrangements for analysis. Later, the data were subjected to RFM analysis with Python programming language. In the last stage, the customer groups’ pasta brand preferences determined according to loyalty dimensions by RFM analysis were analyzed dimensionally by using the SPSS package program. FINDINGS In determining the RFM scores, which is the first part of the research, 18975 customers were analyzed, and the RFM values of each customer were determined by using the Python programming language. Then, based on these values, the RFM scores of the customers were found. Based on these scores, customers are identified according to their scores as “platinum, gold, silver, bronze and risky.” For example, 555 represents the platinum customer group (the best customers), while customers with an RFM score of 111 represent the risky group. The best customers are the customers with the highest purchase frequency and volume. The last part of the analysis is the graphical representation of the relationship between customer segments and pasta brands, presented in Figure 1. In this chart, the correlation between customer segment category (row) and pasta brands (column) can be evaluated according to the proximity of the profile points on the chart. The shortness of distance between the pasta brand and customer segment shows the strength of the relationship. CONCLUSION Competition is increasing in today’s retail industry. The way retailers can survive and increase their profitability in this competitive environment is to know their customer base well. Identifying customer groups can be achieved by applying the right segmentation strategies, but customer segmentation based on demographic factors alone is not enough today. Therefore, retail businesses need techniques such as RFM analysis that they can easily apply. Considering that statistical modelling can be more expensive than the RFM method due to the need for well-trained experts and software costs, RFM can be considered an economical and generally trustworthy tool (Zabkowski, 2016). Using the data in customer databases owned by retail businesses in their segmentation efforts and making them meaningful with RFM analysis techniques will enable a clearer and healthier customer segmentation. Thanks to RFM analysis, customers can be segmented by looking at how often they buy, when they last visited the store, how much they spent, and how appropriate names can be given to these segments. In this way, the promotion efforts to be applied to customers will be more effective. However, segmenting customers into specific groups will not be sufficient. In this way, the promotion efforts to be applied to customers will be more effective. However, segmenting customers into specific groups will not be sufficient. Answering the question of which products and which brands of these products are preferred by these segments is essential for retail businesses, especially in deciding on product diversity. Therefore, as in our study, the combination of RFM and correspondence analysis can enable an effective customer-product and brand match. This study shows how customer segmentation can be done for retail companies by analyzing sales data using two different analysis types. The data used in the study include customer loyalty cards. The study also contributes to the fact that customer segmentation decisions based solely on customer loyalty cards may cause wrong results, and it also shows that customer segmentation based on scientific analysis can provide more detailed information to company managers.