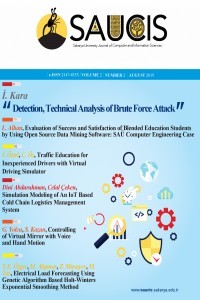
Sakarya University Journal of Computer and Information Sciences
Yazarlar: Burcu DEMİRELLİ OKKALIOĞLU
Konular:Bilgisayar Bilimleri, Bilgi Sistemleri, Bilgisayar Bilimleri, Yapay Zeka
DOI:10.35377/saucis.03.02.714969
Anahtar Kelimeler:Dynamic k,Significance-weighting,Collaborative filtering,Accuracy,Coverage
Özet: Recommender systems have become more and more popular in online environments in recent years. Although different approaches are introduced to build a powerful recommender system, collaborative filtering is one of the most used approaches in the recommender systems. Yet, researchers still introduce new methods to improve prediction performances in collaborative filtering. k nearest neighbor algorithm is one of the most dominant and prevalent one in collaborative filtering. The underlying approach behind it is to select a predefined k neighbors for an active user among all users. In the traditional algorithm, the value of k is constant and is determined before the prediction process. Recently, scholars proposed to use dynamic k neighbor selection for each user. Inspired from this work, we propose to improve prediction performance, accuracy and coverage, of collaborative filtering systems under k nearest neighbor approach. We first propose that users who rate the target item should become nominees for dynamic k neighbor selection instead of all possible users whose similarities can be calculated. The similarity calculation is the most crucial point of the k nearest neighbor algorithm. Furthermore, we also propose to use the significance-weighting approach in addition to the traditional Pearson correlation coefficient when identifying the best dynamic k neighbors for each user. The experimental results on the two well-known datasets show that the prediction accuracy and coverage improve in the dynamic k neighbor selection method by selecting neighbors among users who rated the target item and introducing the significance-weighting factor into the neighbor selection phase to find more eligible neighbors.