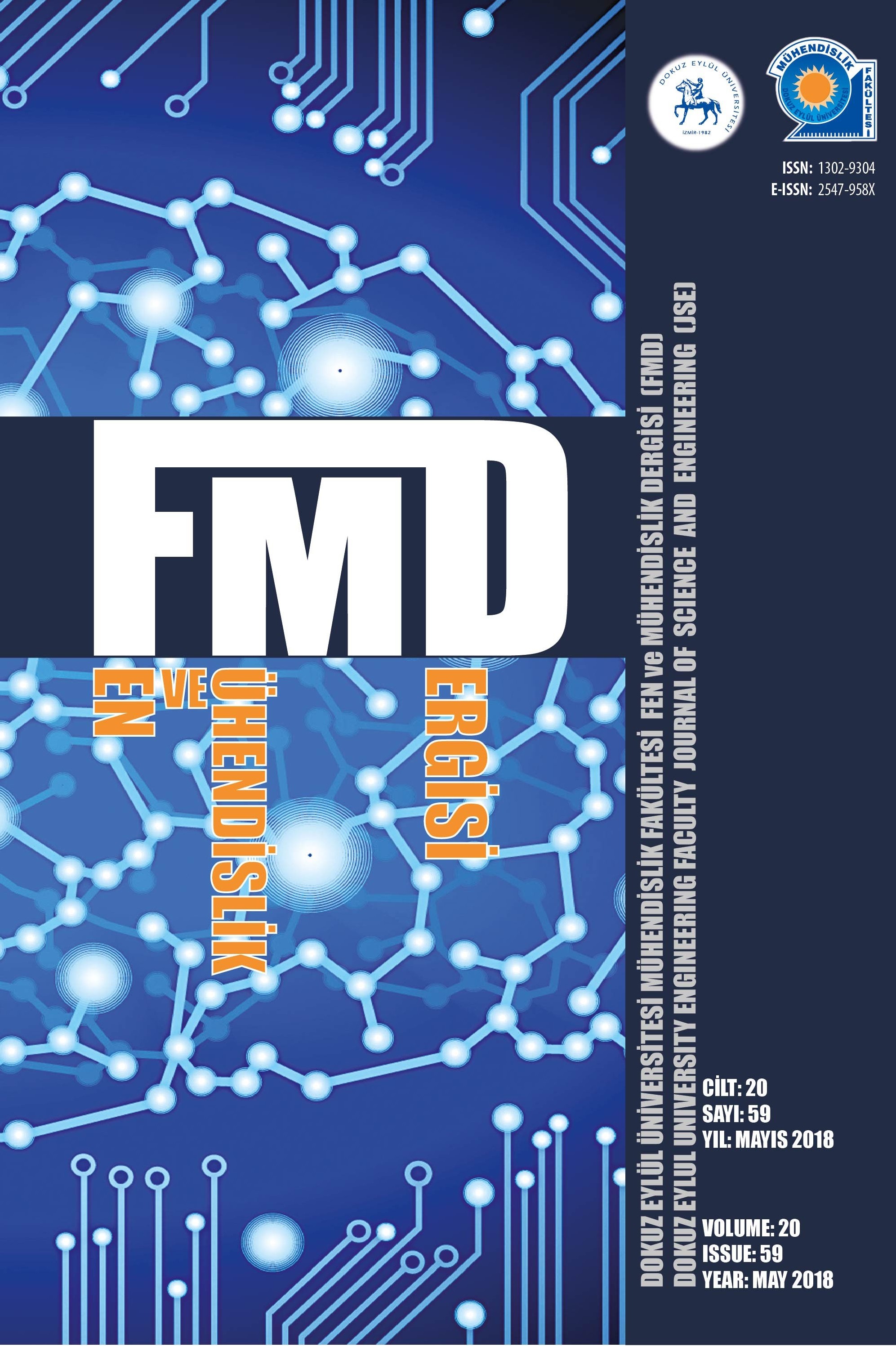
Dokuz Eylül Üniversitesi Mühendislik Fakültesi Fen ve Mühendislik Dergisi
Yazarlar: Volkan KIRANOGLU, Göksu TÜYSÜZOĞLU, Elife ÖZTÜRK KIYAK
Konular:Mühendislik, Ortak Disiplinler
DOI:10.21205/deufmd.2021236828
Anahtar Kelimeler:Active Learning,Classification,Crime Detection,Random Sampling,Semi-Supervised Learning,Self-Learning
Özet: In line with technological developments, machine learning/data mining studies have significantly scaled up in crime analysis. The prediction of crime occurrences, the detection of the spatial/temporal distribution of the criminal cases, forecasting the type of crime are some of these study areas. By taking crime data resulting from a substantial increase in crime rates into consideration, unlabeled data can be utilized to enhance exploring the patterns of crime for future events or to make crime-related predictions easily. Therefore, in this study, active learning, self-learning, and random sampling techniques are applied to predict the outcome of criminal searches in England using the police data of 2019. According to the experimental analysis, active learning outperforms its counterparts using its entropy-based smart selection strategy data in case there is little labeled data.